Understanding Image Datasets for Classification: A Comprehensive Guide
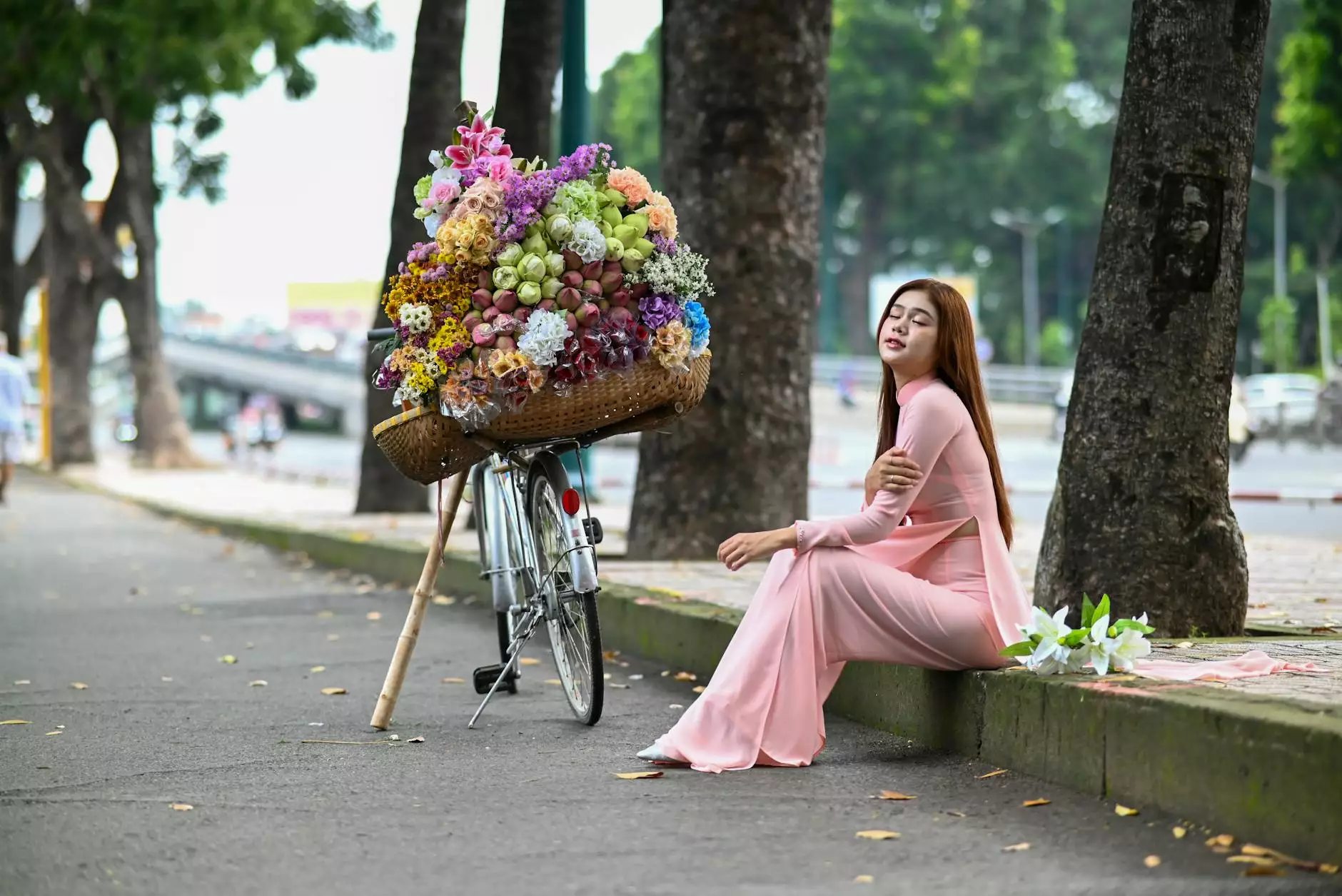
The digital age has seen an unprecedented rise in the need for precise and efficient data classification, particularly in the realm of images. Organizations across various industries are increasingly reliant on image datasets for classification to harness actionable insights from visual data. This guide delves into the significance of image datasets, the processes involving data annotation, and how platforms can streamline this essential task.
What are Image Datasets?
Image datasets are structured collections of images used for a variety of purposes, particularly in training machine learning models. These datasets are integral to tasks such as:
- Object Recognition: Identifying objects within images.
- Facial Recognition: Classifying and recognizing human faces.
- Scene Understanding: Analyzing and interpreting the context within images.
Each dataset comprises images that have been meticulously annotated, meaning they have been labeled and categorized to aid in the training of machine learning algorithms. The quality and richness of these datasets can greatly influence the performance of predictive models and applications.
The Importance of Quality Annotation
Annotation is a pivotal process in the development of image datasets for classification. It involves tagging images with information that the algorithms will use to learn. The precision of this annotation directly impacts the model's ability to classify images correctly.
High-quality annotation includes:
- Accurate Labeling: Ensuring that every image is labelled correctly.
- Comprehensive Coverage: Including a wide variety of examples to train the model effectively.
- Consistent Format: Maintaining a uniform standard for all annotations to minimize confusion during the training phase.
Moreover, incorrect or inconsistent annotations can lead to biases in machine learning models, resulting in poor performance and unreliable predictions.
Challenges in Data Annotation
Despite its importance, data annotation is fraught with challenges, such as:
- Time Consumption: Annotating large datasets is labor-intensive and can take substantial time to accomplish.
- Scalability Issues: As datasets grow in size, maintaining consistent annotation quality becomes increasingly difficult.
- Human Error: Manual annotation is prone to mistakes, which can compromise the integrity of the dataset.
To address these challenges, companies are increasingly adopting advanced data annotation tools and platforms that leverage artificial intelligence to enhance the speed and accuracy of the annotation process.
How Keylabs.ai Enhances Data Annotation
Keylabs.ai is at the forefront of revolutionizing how organizations approach image datasets for classification. With a focus on providing intuitive and efficient data annotation solutions, Keylabs.ai offers a robust platform that caters to the evolving needs of various industries.
Data Annotation Tool Features
Keylabs.ai’s data annotation tool is equipped with numerous features designed to streamline the annotation workflow:
- Automated Annotation: Leveraging AI to pre-label images, saving precious time while maintaining accuracy.
- User-Friendly Interface: A simple and intuitive design that enables teams to collaborate efficiently, reducing the learning curve.
- Quality Control Mechanisms: Features that allow for easy review and correction of annotations, ensuring high integrity of data.
Data Annotation Platform Benefits
The data annotation platform provided by Keylabs.ai allows enterprises to manage their annotation projects more effectively:
- Scalability: Easily scale projects without the burden of hiring additional personnel.
- Cost Efficiency: Save on labor and time costs while improving the quality of annotations.
- Integration Capabilities: Seamlessly integrates with existing workflows and machine learning pipelines.
The Role of Image Datasets in Machine Learning and AI
Machine Learning (ML) and Artificial Intelligence (AI) have transformed numerous industries, and their success is heavily reliant on the quality of the data utilized, especially that of image datasets for classification. The role of these datasets can be summarized in the following key areas:
1. Improving Accuracy
Training ML models with high-quality image datasets significantly enhances the model's ability to understand and classify new images accurately. The more comprehensive and representative the dataset, the better the performance of the model.
2. Enabling Innovation
Broad image datasets facilitate the experimentation and implementation of new algorithms, driving innovation in areas such as autonomous vehicles, healthcare imaging, and security systems.
3. Understanding Consumer Behavior
Businesses leverage image datasets to analyze consumer behavior and trends. By classifying images shared by users on social media, businesses can tailor marketing strategies and improve customer experiences.
Best Practices for Creating Image Datasets for Classification
To develop effective image datasets, consider the following best practices:
- Define Clear Objectives: Understand what you want to achieve with the dataset, including the types of images you need and the annotations required.
- Diverse Image Collection: Include a variety of images that represent all possible scenarios your model will encounter.
- Continuous Updates: Regularly update your dataset to include new images and annotations, ensuring the model remains effective over time.
The Future of Image Data and Classification
As technology evolves, the future of image datasets for classification promises even more sophistication. Advanced machine learning techniques such as deep learning are pushing the boundaries of what is possible in image classification.
Furthermore, with the emergence of more powerful AI tools and platforms, the annotation process is expected to become even more efficient, opening doors for innovations that we can't yet fully envision.
Conclusion
In conclusion, the significance of image datasets for classification cannot be understated in our increasingly data-driven world. The insights derived from effectively annotated images can redefine industries, enhance customer experiences, and drive technological advancements.
By utilizing reliable tools and platforms such as Keylabs.ai, organizations can better navigate the complexities of data annotation, ensuring that their image datasets are of the highest quality and can serve the intended purpose without bias or error.
Investing in the right data annotation tools and platforms is not just an operational decision but a strategic one that can position businesses for success in the digital landscape.