Understanding Bounding Boxes in Data Annotation
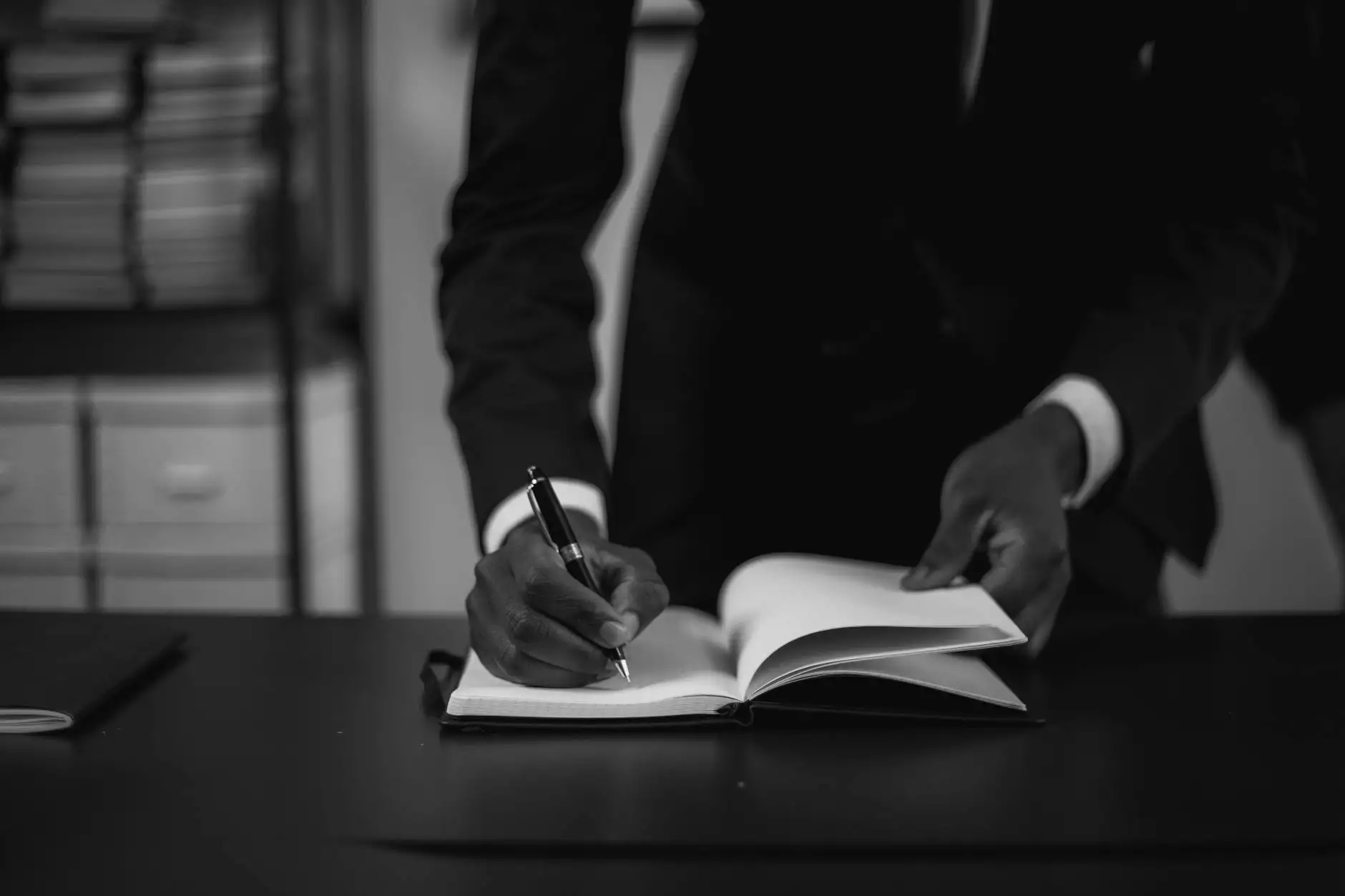
In the realm of data annotation, the term bounding boxes plays a pivotal role. These simple rectangles are not just basic shapes; they are essential tools that form the foundation of many computer vision applications. From detecting objects in images to training machine learning models, bounding boxes allow us to delineate areas of interest within complex visual data. In this article, we will delve deep into the significance of bounding boxes, examining their definitions, applications, and the best practices for utilizing them effectively in your data annotation platform.
What Are Bounding Boxes?
A bounding box is defined as a rectangular box that is used to draw a boundary around an object or area of interest within an image. These boxes are characterized by their coordinates, typically represented by the top-left and bottom-right corners or by the center point alongside width and height. The simplicity of this representation allows for quick and efficient identification of objects.
Applications of Bounding Boxes
Bounding boxes find applications across various domains, making them a critical component in any data annotation tool. Here are some key applications:
- Object Detection: In computer vision, bounding boxes are used to identify and locate objects within images, allowing algorithms to classify and understand visuals.
- Image Segmentation: While bounding boxes provide a coarse boundary, they serve as preliminary steps to more refined segmentation techniques.
- Video Analysis: In video data, bounding boxes track moving objects frame by frame, essential for activities such as surveillance and behavior analysis.
- Facial Recognition: Bounding boxes can isolate faces in photos, making it easier for algorithms to analyze facial features for recognition scenarios.
Why Are Bounding Boxes Important in Data Annotation?
Bounding boxes significantly enhance the quality and reliability of datasets used in training machine learning models. Here are several reasons why they are so crucial:
- Improved Accuracy: By clearly defining areas that contain objects of interest, bounding boxes improve the accuracy of detection algorithms.
- Simplified Annotation: Their straightforward nature makes it easier for annotators to define and label images, thereby expediting the data preparation phase.
- Scalability: When large datasets are involved, stable bounding box definitions ensure consistent annotations across numerous images, contributing to reliable machine learning outcomes.
- Facilitating Supervised Learning: Bounding boxes create precise training sets for supervised learning models, directly impacting the effectiveness of the output.
Best Practices for Using Bounding Boxes
Utilizing bounding boxes effectively in your data annotation platform necessitates following best practices to ensure high-quality annotations:
1. Ensure Precision in Annotation
When creating bounding boxes, it is essential to draw them as closely as possible around the object of interest. This reduces errors and improves model training accuracy. Use precise tools that allow fine adjustments to box dimensions.
2. Maintain Consistency
Consistency is fundamental when annotating images. All annotators should adhere to the same set of guidelines regarding what constitutes a bounding box. This includes considerations for partially obscured objects or multiple objects in close proximity.
3. Utilize Tools and Software
Consider using advanced data annotation tools that offer features specifically designed for bounding box creation. These tools can enhance productivity and ensure higher quality through user-friendly interfaces and intelligent suggestions.
4. Review and Validate Annotations
After completing the annotations, it is crucial to have a review process in place. Peer reviews can highlight inconsistencies and errors that may have been overlooked, thereby ensuring data integrity.
The Future of Bounding Boxes in Machine Learning
The role of bounding boxes is evolving alongside advancements in machine learning and computer vision technologies. Here are some trends to watch:
1. Integration with Advanced Algorithms
As algorithms become more sophisticated, bounding boxes will continue to play a significant role in both training datasets and performance evaluation.
2. Transitioning to 3D Annotations
With the rise of virtual and augmented reality, techniques for 3D annotations are developing, yet bounding boxes will remain foundational, allowing a bridge from 2D annotations to more complex scenarios.
3. Automation in Annotation
Automation tools are being developed that utilize machine learning to automate the bounding box creation process. While automation will not eliminate the need for human oversight, it may significantly reduce the time spent on initial annotations.
How Keylabs.ai is Leading in Data Annotation
At Keylabs.ai, we understand the critical role that bounding boxes play in data annotation, particularly in enhancing the capabilities of our data annotation tools and platforms. Our commitment to quality and precision ensures that your data is annotated correctly, meeting the highest industry standards. Here’s how we stand out:
1. Advanced Annotation Tools
We offer state-of-the-art tools that streamline the annotation process, incorporating features such as:
- Real-time collaboration for team annotation efforts.
- Intelligent box-drawing assistance that recommends box sizes based on image analysis.
- Customizable annotation guidelines that align with your unique project requirements.
2. Expert Annotation Team
Our skilled team of annotators is trained to deliver high-quality results. They understand the nuances of proper bounding box creation, ensuring data is annotated with the right context.
3. Quality Assurance Protocols
Quality is non-negotiable at Keylabs.ai. Our robust review processes guarantee that all bounding boxes created are precise and reliable, ultimately ensuring that your machine learning models perform effectively.
Conclusion
In summary, bounding boxes are indispensable in the data annotation space, forming the backbone for countless applications in computer vision and machine learning. By adhering to best practices and utilizing advanced tools, organizations can harness the full potential of bounding boxes to improve accuracy and efficiency in their projects. At Keylabs.ai, we pride ourselves on our expertise in delivering exceptional data annotation services, ensuring that every bounding box adds value to your datasets. Choose Keylabs.ai for your next data annotation project and experience the difference quality makes!